Machine Learning Workflow for a New Bamboo Construction Methodology
Bamboo as a natural building material has a lot of variation due to the natural way it grows. This characteristic of bamboo can make it difficult, time confusing and limits the usage of the material to only expert craftsmen.
There is an opportunity here to apply Artificial Intelligence to the practice. Training a model to predict the bending possibilities of a single culm of bamboo makes the design process and selection of bamboo culms seamless. By using machine learning we can manage the unpredictability of bamboo and also create a more precise design and push the material into mainstream construction usage.
Universal Goal of Sustainable Material & Construction
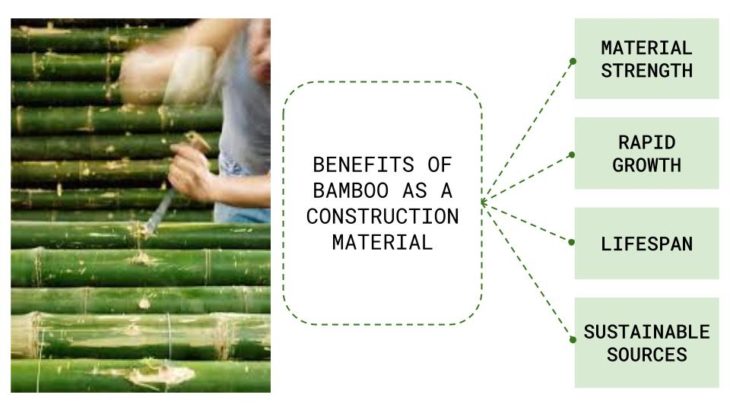
Bamboo has a huge potential as a sustainable alternative to building material. It is fast to grow and does not not require much resources. It also has strong structural potential when used in the right way.
Precedents – Current Bamboo Designers & Structures
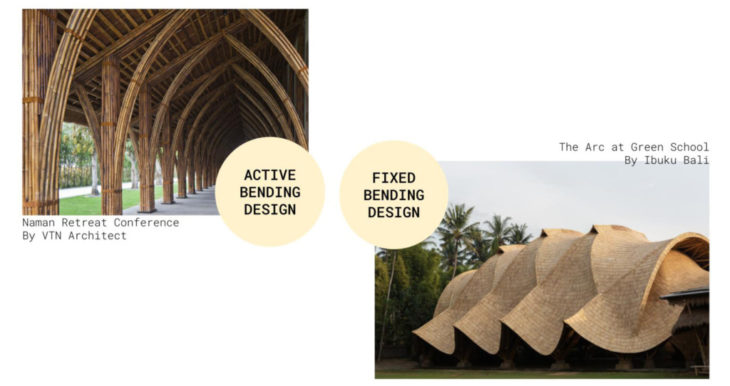
The Bamboo architecture works that we currently see being built mainly follow 2 different methods of manipulating bamboo.
Working with Bamboo – Part 1
The current bamboo construction method follows a top down approach to design. The architectural and structural design of the bamboo building is separate from the process of cultivating, preparing and shaping the bamboo culms for its final purpose.
Working with Bamboo – Part 2
Taking into account that bamboo is a natural material that will grow to have many varying culms characteristics, the harvested bamboo will have to be manipulated in multiple ways to fit the pre-designed structure.
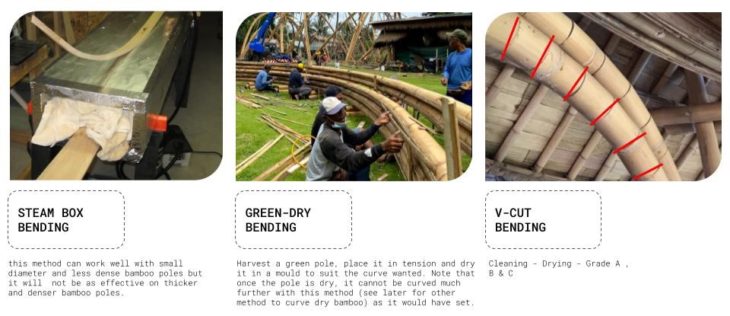
Objective – The thesis looks at incorporating machine learning into the process of building in a holistic way. We aim to train a model to give us the predicted bending capabilities of each unique culm in a particular yearly harvest and this in turn will inform the design, creating a bottom-up form finding process.
Dataset creation is key to training and improving a ML model.
- Placeholder of bamboo harvest is created with a parametric model that mimics bamboo behavior.
- Eventually a real dataset of collected bamboo can replace this digitally made dataset in order to improve the accuracy of the trained model.
Anatomy & Species
To create a ML model that performs well, we need a parametric dataset that best reflects the true behavior of bamboo bending. Firstly we need to understand the anatomy that effects the movement and growth of bamboo.
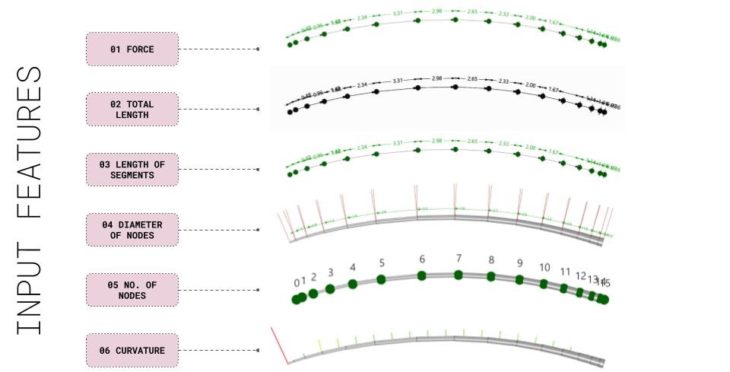
There are 6 main features that influence the behavior of the culms.
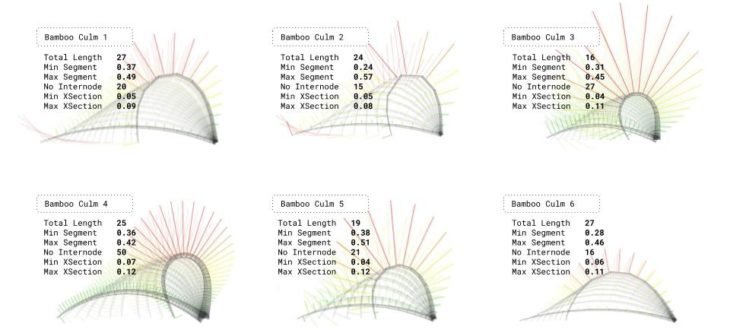
The 6 features are allowed to iterate to create the dataset. Diversity in the parameters will avoid the model from being biased.
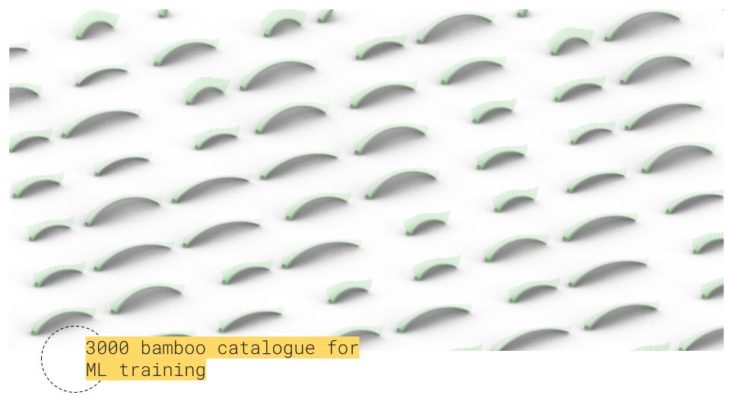
The dataset created from the parametric model contains many variations of bamboo possibilities, ideal and non-ideal ones for construction. The model will then be able to train on a diverse set of information.
We decide to test both a shallow learning model as well as a deep learning model.
MODEL TRAINING 1
Shallow Learning – Linear Regression
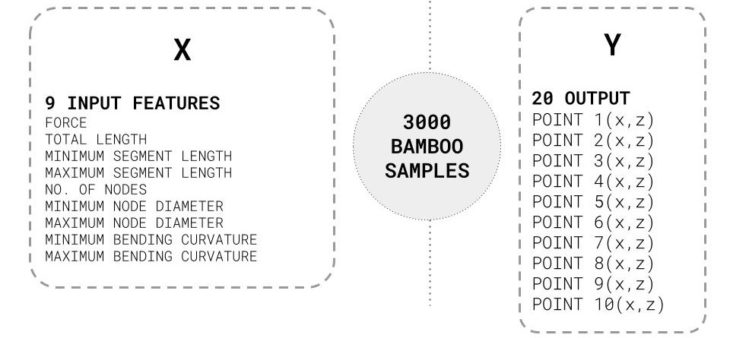
The first training was using a linear regression model, with 9 inputs and 20 outputs. The inputs are based on the parametric model and design space mentioned earlier where as the outputs are the x and z coordinates of the points of the curve to the corresponding inputs.
Model 1 Results & Accuracy
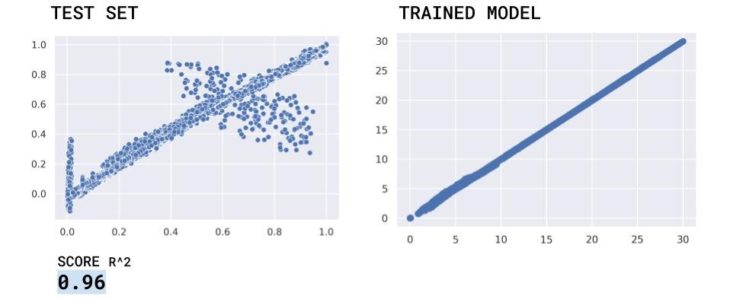
We get a pretty accurate result with a sore of .96 for the linear regression model.
MODEL TRAINING 2
Deep Learning – Artificial Neural Network
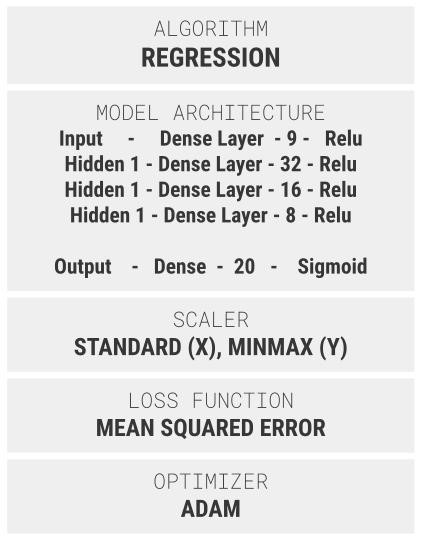
For the deep learning model we used an regression artificial neural network. Using a standard scaler for the x features and a min-max scaler for the y features, mean squared error for the the loss function and Adam for the optimizer. We noticed that these gave us the best results.
Model 2 Results & Accuracy
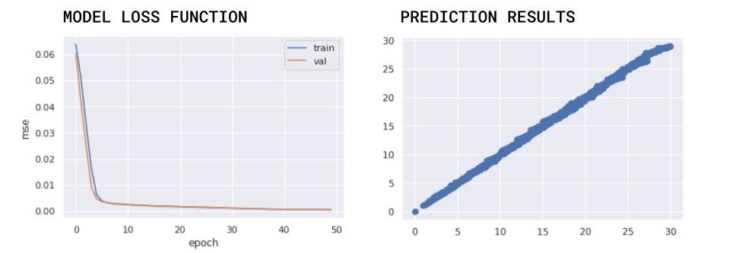
The prediction results are comparable between the shallow and deep learning models. We decided to move ahead with the shallow linear regression model. We learned from this process that a simple model sometimes is sufficient to achieve our goals and therefore better simple solution.
Geometry in Rhino – HOPS
For this we used the hops component that allows us to bring in external functions to grasshopper. Using python to create an app through flask we are able to use the trained machine learning model in grasshopper. Here, we see the hops component taking the inputs from a csv file and generating the coordinates of the points to predict the bending.
Validating the Predicted Geometry
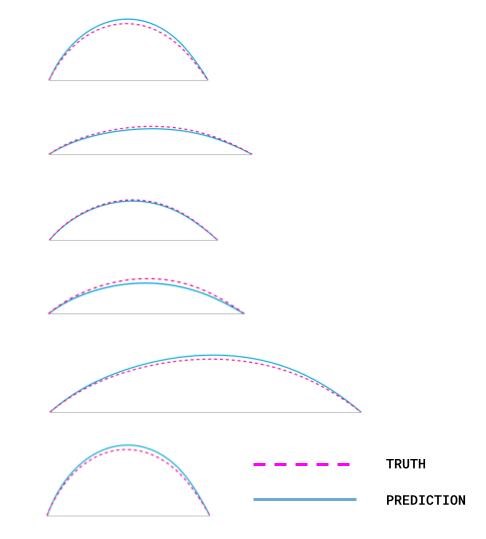
We compare the actual bending moments to the predicted bending moments generated through hops and evaluated the deviation between the two. The dotted magenta shows the actual results and the blue shows the predicted ones. We can see that the predictions are not far off from the validating set.
Moving forward to phase 2, we can then begin to explore the bamboo form finding with other computational methods. Taking into considerations the bending/structural capabilities of the harvested culms we can iterate designs based on other spatial or environmental objectives.
‘Advanced Bamboo Workflow’ is a project of IAAC, Institute for Advanced Architecture of Catalonia developed in the Masters of Advanced Computation for Architecture & Design 2020/21 by Students: Marissa Ridzuan & Amar Gurung and Faculty: David Andres Leon