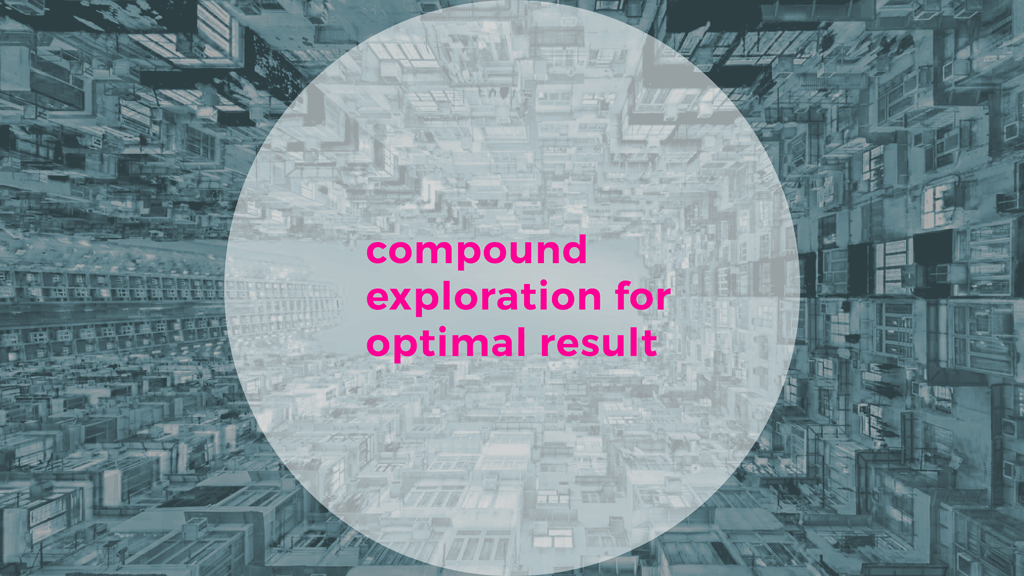
CONTEXT
TRANSLATION INTO SPATIAL STRATEGY
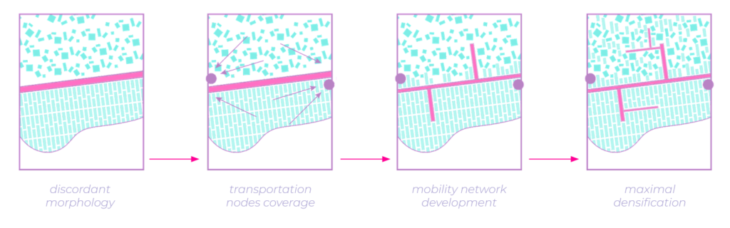
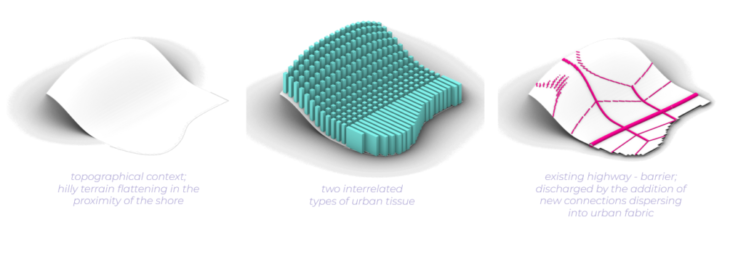
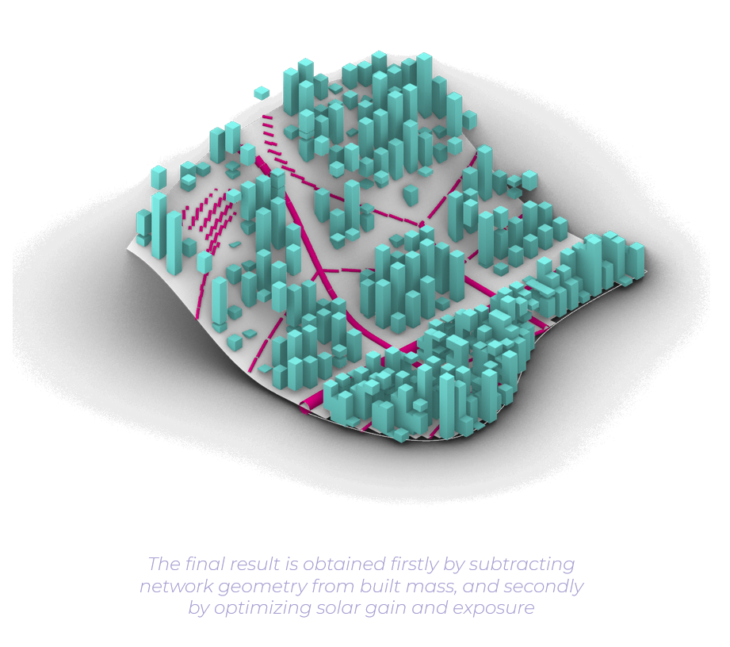
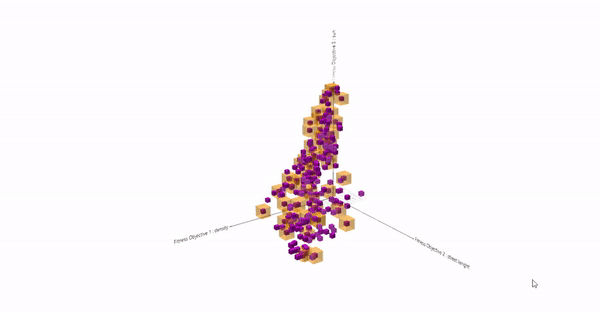
COMPUTING VARIATIONS AND UNDERSTANDING PERFORMANCE
Considering the structure of Wallacei analisis, in order to generate evolutionary designs for the city of Hong Kong 3 main objectives were considered: increase of density (measured by the construction volume), connectivity (measured by the length of the streets of an abstract mobility network) and solar gain (measured by occlusion factors of the shadows in the terrain). It is important to point out how two of the three main objectives have clashing outputs, them being increase of solar gain and increase of density, characteristics which provided the analysis with interesting results.
Setting the 3 main goals, also called fitness criterias in the evolutionary lexic, the Wallacei plugin ran considering 20 individuals throughout 40 generations. This means that 800 different designs for optimizing the urban tissue of Hong Kong were generated, each one evolving in certain aspects.
F01 – density || F02 – street length || F03 – solar gain
When looking at the results produced by Wallacei, a first effort was made to understand how the exploitation of each fitness criteria could modify the results and create very different geometries. For this purpose, the 3 extreme models were analyzed: one considering the maximum density to be reached, the second one with the highest index of street length and the third one considering the maximum of solar gain in the urban tissue.
Considering these results, it is possible to observe the clashing nature from 2 of the 3 fitness criteria chosen, them being solar gain and density. Looking at the standard deviation graphics and the diamond charts, it becomes clear that whenever there are high indexes for solar gain, density is highly compromised and vice versa. Although, it is also interesting to notice that the solar gain is the most influential factor, also jeopardizing the street length fitness criteria when pulled to the extreme.
CLUSTERING GENERATIONS
Thus, having the understanding of how influential are the fitness criteria and in order to select possible designs which could represent a satisfactory result for all the objectives, it was chosen to analyze the Pareto front¹ results. These results were then clustered by average-linkage².
Within the 4 clusters that were created, one individual was chosen to have the design analyzed. When plotting these options side by side, guided also by the generation of the diamond charts, it was possible to identify one design which had a balanced distribution of the fitness criteria to be reached, which is possible to observe in the image below.
As a conclusion from the analysis, it would be preferable to redefine the calculations of the fitness criteria in a more detailed form and run the analysis considering more generations, since the optimizations results are present in the standard deviation graphics but do not reach a stability yet. This can also be seen in the genome created for each individual generated in the simulation, where it is possible to observe a random genome going towards a more organized one, as generations of design pass.
¹ Pareto efficiency or Pareto optimality is a situation where no individual or preference criterion can be better off without making at least one individual or preference criterion worse off or without any loss thereof.
² Clustering tries to find structure in data by creating groupings of data with similar characteristics. Average-linkage is where the distance between each pair of observations in each cluster are added up and divided by the number of pairs to get an average inter-cluster distance.