INTRODUCTION
Land has been seen as a categoric status regarding spatial planning, but land has a multifunctional value that is oftenly not recognized. This has lead to an indiscriminate consumption of land, creating unbalanced territories with environmental and social issues.
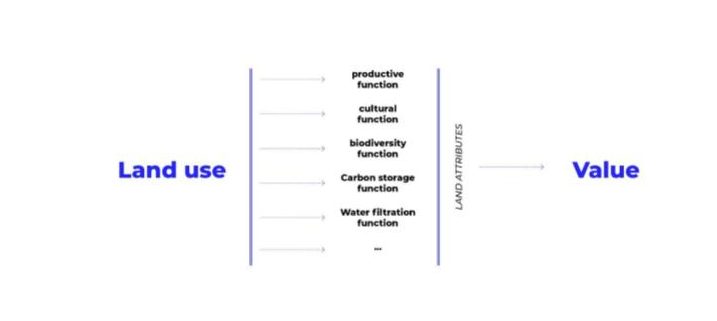
Land use functions
NEW LAND CATEGORIZATION
In order to understand better land processes, two indicators were chosen in a specific context, Vitoria-Gasteiz city. Taking into account the actual categories of land use, the offers and the demands of land of Co2 sequestration adn Food production were measured.
PROCESS
Taking into consideration the data analysis provided, two clustering methods were chosen in order to understand the hidden patterns of the landscape: Agglomerative and K-means Clustering.
CLUSTERING EVALUATION
Different number of clusters were tested with different types of clustering. The 5 clusters represented better the territory, and the agglomerative clustering showed less standard deviation inside the clusters. This allowed to understand that more similar attributes were within this method inside each cluster.
DEPLOYMENT
The measurement of the territory and the type of clusters were deployed through bookeh in order to build an interactive interface.
LAND AI: UNSUPERVISED LEARNING FOR CLUSTERING LAND FUNCTIONS is a project of IAAC, Institute for Advanced Architecture of Catalonia developed at Master in City & Technology in 2021/22 by student: Adriana Aguirre Such and faculty: Angelos Chronis, Nariddh Khean, Serjoscha Duering.