L.U.R. is a knowledge-based framework for innovative practice based on measuring land resilience. Through a data-driven approach, the framework proposes a responsive taxonomy that allows an understanding of land’s transitional dynamics. The framework offers a continuous feedback loop methodology to reclassify land units from a systemic socio-ecological perspective to recognize land’s multifunctional value and enrich decision-making processes affecting land.
LAND USE VS. LAND FUNCTIONS
We are starting to understand that the future is defined by its uncertainty. Therefore, our solutions should embrace that complexity to respond to current and future challenges. However, what is certain is that we are running out of land. The more we are, the more our access to resources improves, the more we use them. Nowadays, humanity uses resources at a rate 50% faster than can be regenerated by nature, and the global demand is expected to increase dramatically in the coming years. The idea of the urban process understands urban settlements beyond its boundaries, where the urban is considered not only the city itself but also the space that sustains it, such as the land for food production. Those urban processes take in Europe 2500 more hectares of land than required for the built-up areas. When urban processes change, the land-use changes and vice versa.
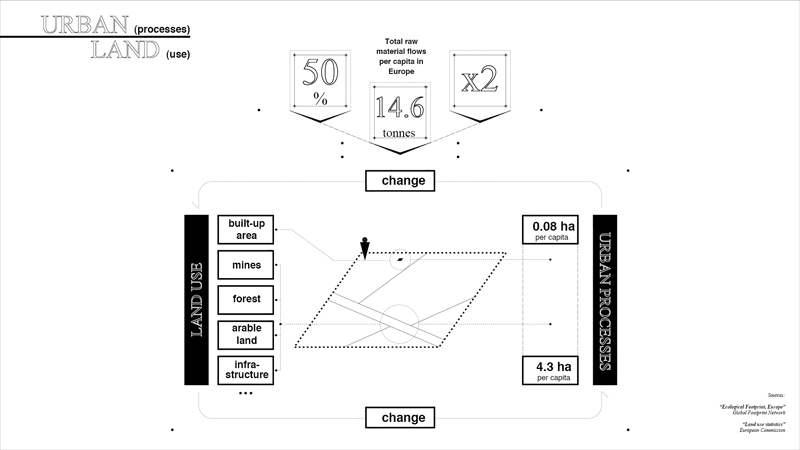
Urban Processes & Land Usue
On top of this extractive understanding of the land, as a resource provider, the global capacity of nature to regenerate is limited. To satisfy Europe’s current demand, 3 planets Earth would be needed. Nature cannot keep the human rhythm to process all the unintended outcomes of urban processes. Land provides a multiplicity of functions simultaneously, constantly overlooked and ignored: It produces food but also stores carbon, provides habitat for biodiversity, and many more. These functions are linked and compose an interconnected system. The land has more value than the one that is commonly acknowledged.
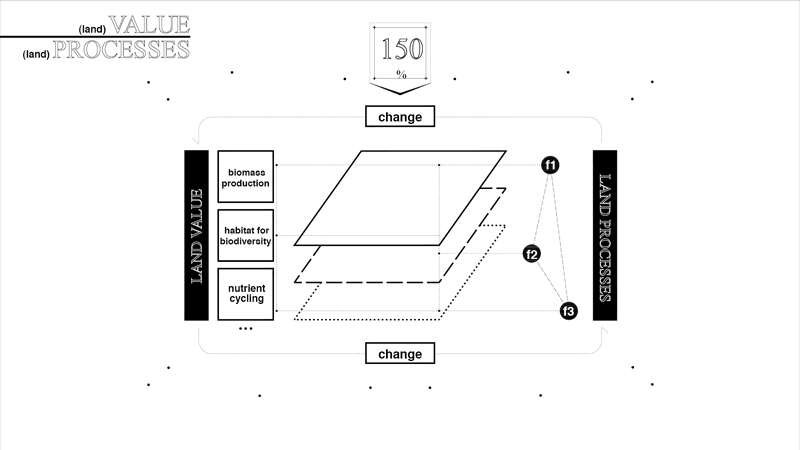
Land processes, Land Functions & Land Values
THE LAND USE ISSUE
Land-use fragments this system. It only considers the socioeconomic functional dimension of the land, bounding and constraining land into categories. The categories of land use and the spatial fragmentation that represents these categories also affect decision-making processes. By dividing land into uses, decision-makers tend to focus on its area of action, enhancing a specific mono functionality. As a consequence; silver bullet solutions are proposed, tending to neglect land’s multifunctional condition and ignoring the multiple values that land provides. As we progress toward future conditions, we have shifting challenges, and as a result, we cannot respond to that uncertainty that characterizes the future.
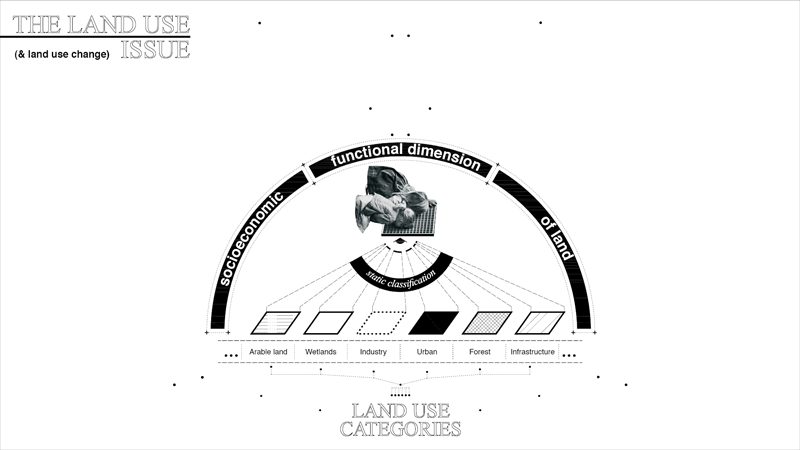
Territorial & Functional Fragmentation
NON SYSTEMIC TERRITORY
From this territorial reading, as a consequence, land is understood from a monofunctional perspective, instead of having a systemic understanding. As a consequence, this reading creates a territorial fragmentation. By acknowledging the interconectedness of land processes, land’s multifunctional value could enrich and coordinate our decision-making processes by evidence through the application of data-driven digital tools.
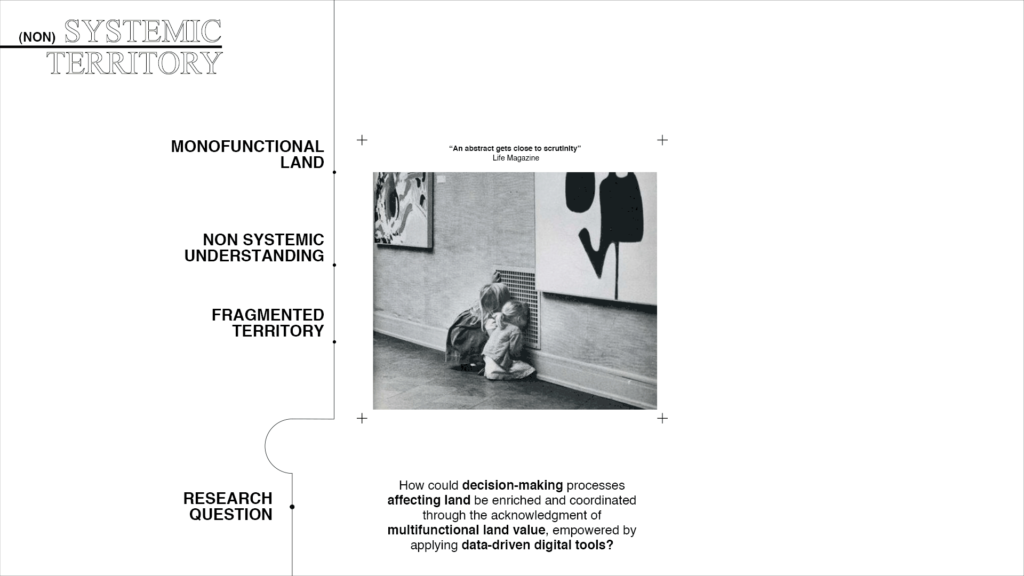
Research Question
We are not having a systemic approach to the territory that should recognize the uniqueness of each part that characterizes it as a system and the lack of fixed identity due to the dynamic condition of the territory itself. We are neglecting that land is a socio-ecological system where humans and environmental subsystems interact. Finally, we are losing the multiple values that land provides because we don’t acknowledge its functions through the evidence to create territorial, dynamic functional assessments.
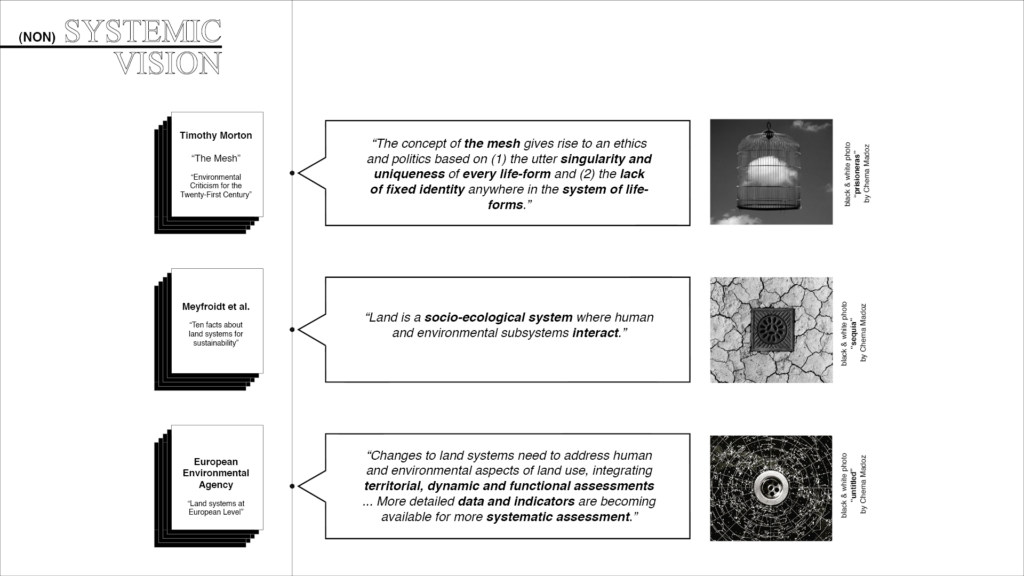
Conceptual Framework
There has been a significant improvement regarding different approaches to recognize land’s value, but at the same time, there is still a fragmented vision subject to land use. These tools are neither systemic nor responsive to the dynamicity that the system creates. Using a data-driven approach, we could respond to that dynamic factor.
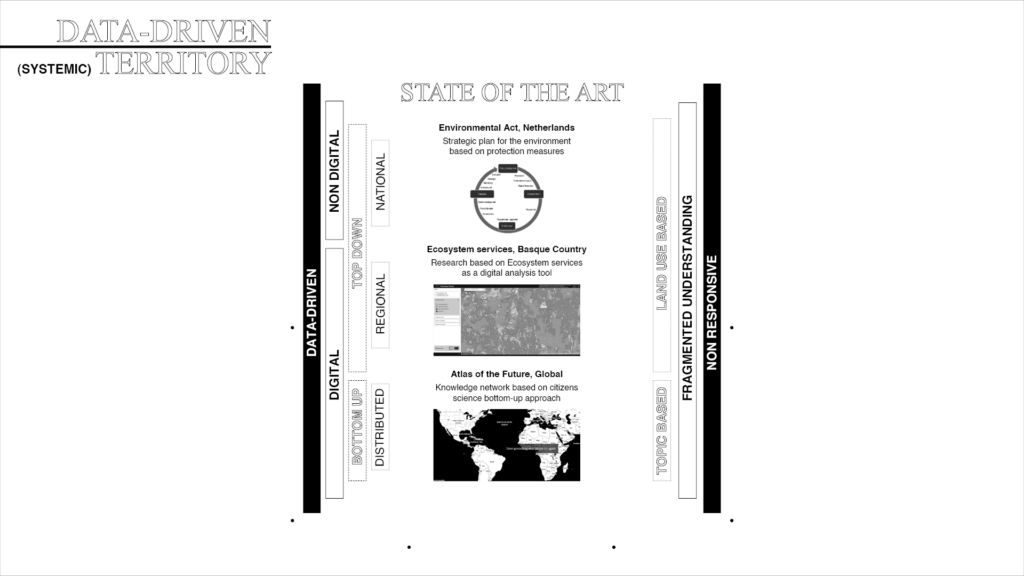
State of the Art
A NEW RESPONSIVE LAND TAXONOMY
We need to move beyond the idea of land use and propose a new taxonomy. Ecosystem services, in that matter, have been recognized as the missing link between society and natural systems and represent this multifunctional dimension. This taxonomy considers the socio-ecological functional dimensions. Measuring and aggregating different ecosystem services enables a new territorial reading based on taxons. The purpose of the new taxons would be not only to recognize the diversity of functions but also the diversity of interactions between these functions.
The new taxons based on multiple values enable a multi-criteria assessment. Consequently, hybrid solutions can be proposed and evaluated by accepting that every solution will have trade-offs, allowing the recovery of a diversity of land functions. As the option value of the land increases, the ability of the territory to respond to uncertainty also increases. By re-understanding land from a socio-ecological perspective, land resilience can be measured and recognize the trade-offs and synergies of our decisions to better plan the urban processes that constantly modify the land.
L.and U.se R.esilience FRAMEWORK
L.U.R framework can simulate and evaluate land resilience through a data-driven approach by creating a responsive taxonomy that allows us to understand the transitional dynamics of the land. LUR decision support system is a knowledge-based communication framework between researchers, policymakers, and spatial planners. The primary users can evaluate new strategies based on socio-ecological indicators, and after their implementation, these interventions can be monitored continuously.
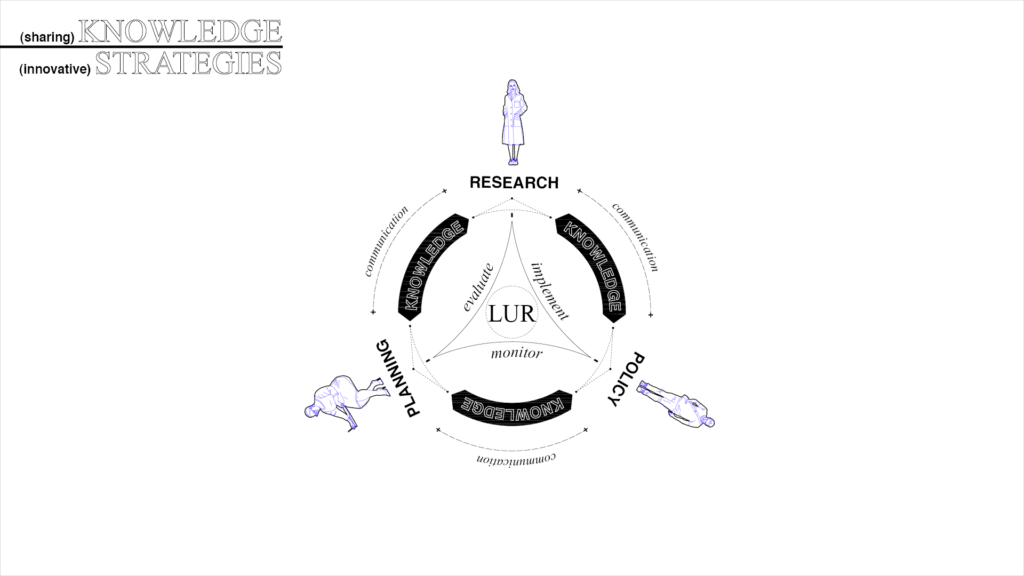
A knowledge-based communication framework
To test this framework, the municipality of Vitoria Gasteiz is chosen in the Basque Country region, in the north of Spain. This municipality, of almost 300 square kilometers, has a great variety of land uses, where only 10% is grey infrastructure. At the same time is characterized by its continuous effort to evolve towards sustainability.
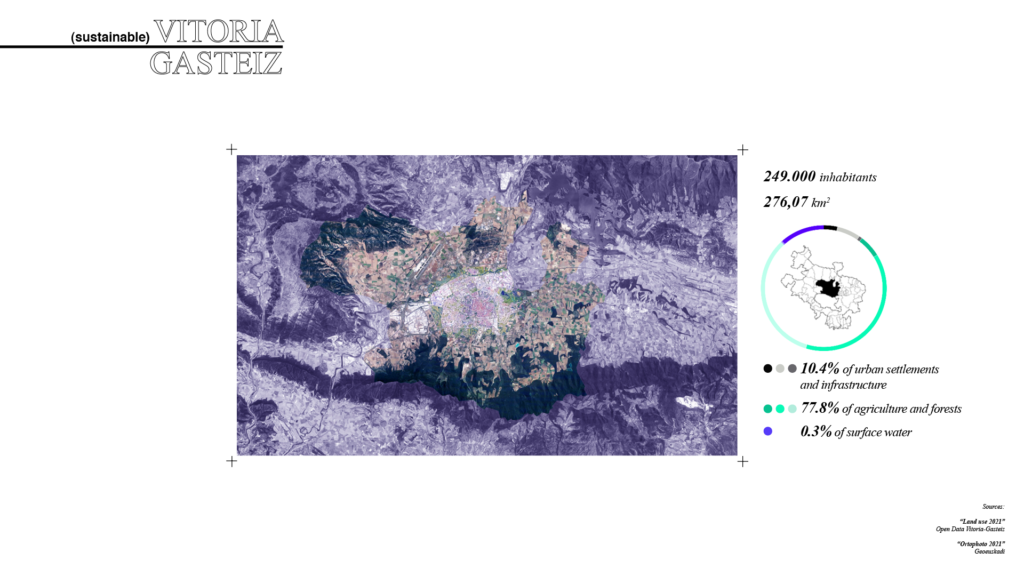
Vitoria Gasteiz as a showcase study
L.U.R METHODOLOGY
The methodology of this framework proposes a continuous feedback loop that starts with a spatial analysis based on Ecosystem services. After, a new taxonomy driven by unsupervised machine learning is created. As a result, the land is grouped depending on the relationship between the functions of the land. From these taxons, hybrid strategies can be proposed and evaluated.
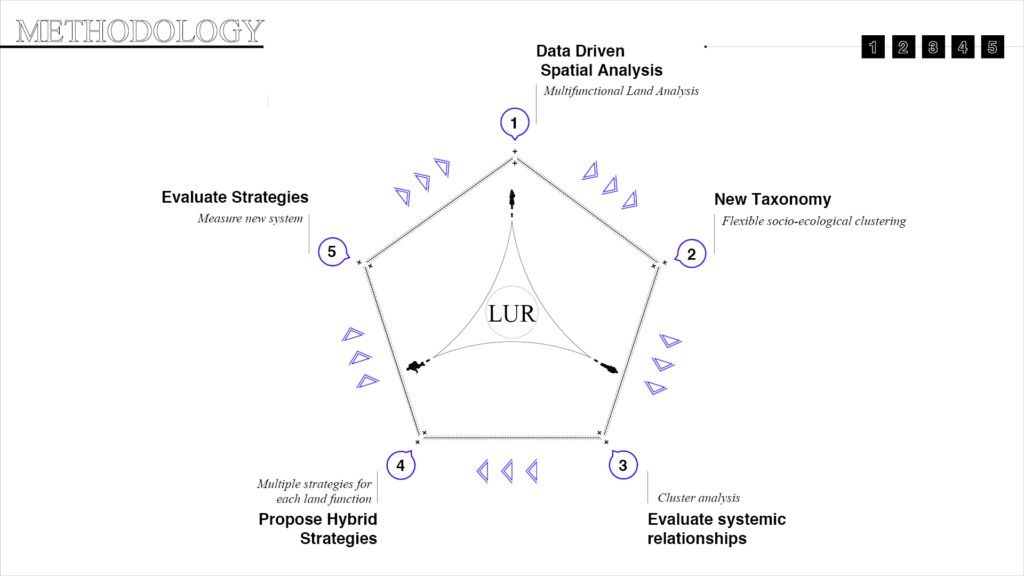
Feedback loop methodology
1.DATA DRIVEN MULTIFUNCTIONAL LAND ANALYSIS
For the first step of the methodology, Soil Fertility, Habitat Maintenance, Carbon Sequestration, and Food Production were measured to create the indicators of the first part of the methodology. These Ecosystem services were selected due to methodological limitations and data availability. Different information was used to calculate the indicators. Global, European, national, and regional sources were used, combining statistical and geospatial data.
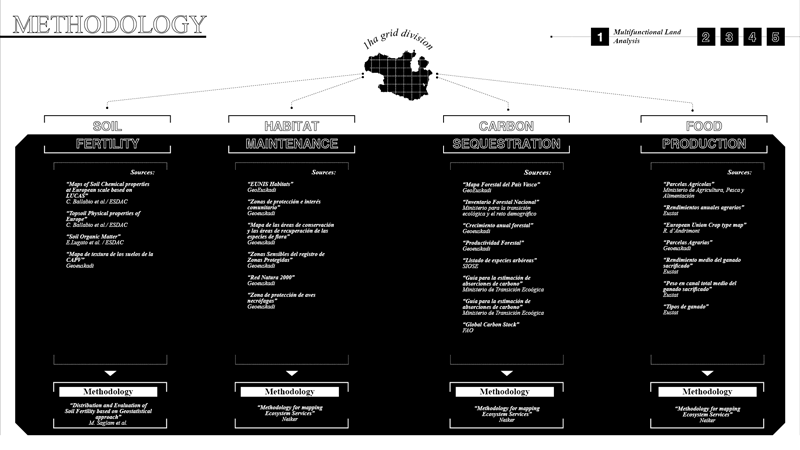
Ecosystem Service based analysis: sources and methods
- The soil fertility index allows scoring the land geospatially. The indicator takes into account macronutrient availability and physical and chemical properties of the soil with the formula below.
- The habitat Maintenance Index scores the land based on the protected areas, the richness of autochthonous vegetation species, and the successional state or ecosystem maturity.
- For the Carbon sequestration, storage in trees and in soils was calculated. Trees sequester different quantities depending on their maturity and the species. For soils, the current carbon stock and the future estimation in the absence of new action were considered. Both procedures allowed spatializing the carbon sequestration in tonnes per hectare per year.
- For the food production, Plant-Based Food, and Meat production were measured. With statistical data, the Plant-Based Food was spatialized only in the arable land, and the meat was spatialized only in the pastures.
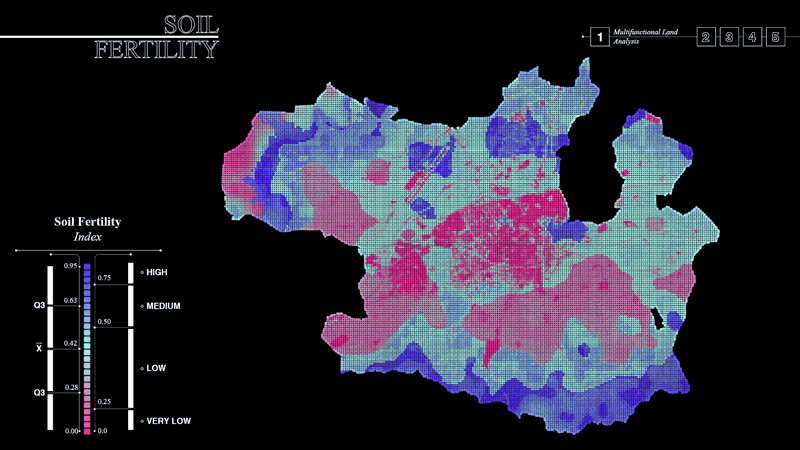
Results
The four results have been divided by quartiles to understand the territory’s performance based on space percentages. In the following images, is possible to see that the distribution of land functions varies in each pixel for each indicator.
- The spatialization of the Soil Fertility in Vitoria allowed understanding that 60% of the area has very low fertility. At the same time, only 10% of the territory has a high one.
- In terms of habitat maintenance, only 5% of the territory has a high score, while 60% has a low one.
- Most of the Carbon in the territory is stored in trees, which represent 20% of the land, but not in a distributed way.
- Regarding food production, 50% of the territory produces between 3 and 10 tonnes, with a very high asymmetry in terms of space.
From this analysis, we can conclude that all indicators show different territorial readings. Specific performance of one indicator doesn’t necessarily mean a similar performance in the other. Therefore, the hidden patterns between these values should be analyzed to understand the systemic relationships between the land functions.
NEW LAND TYPES
In orden to understand these systemic relationshisps the second, third, fourth and fifth part of the methodology are tested.
- First, the four main indicators are aggregated in a flexible grid that allows data resampling. To be able to establish these systemic relationships, an unsupervised machine learning algorithm is applied, K-means clustering, to group the values and create the new taxonomy.
- Once the optimal number of clusters is selected, they are filtered by a fifth data layer, the land use, to include the socioeconomic perspective in this analysis. Clusters can be selected to propose territorial strategies by analyzing the systemic relationships.
- Through the literature review, multiple strategies can be chosen for each cluster. This process allows us to understand the modification of the data values for each indicator.
- With this information, the strategies can be evaluated by comparing them to the current status. By aggregating this new information into the data structure, new data values for the different indicators can be modified. It is essential to acknowledge that these new values should be based on experts to have an accurate evaluation process.
NEW TAXONOMY
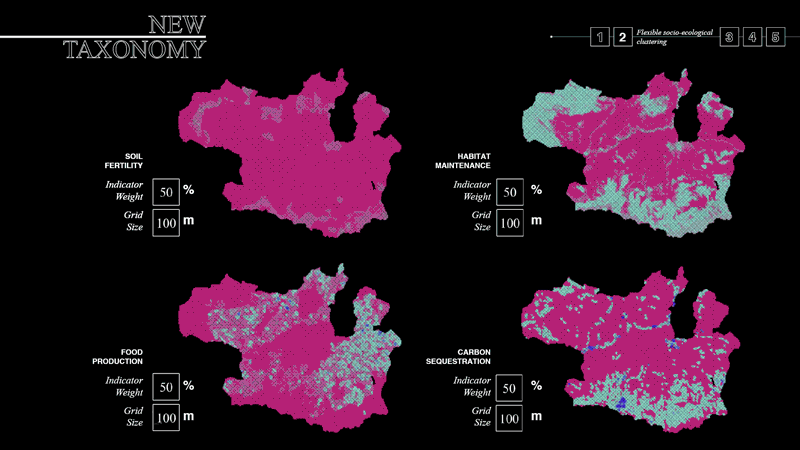
Flexible Socioecological clustering
The socio-ecological grid allows a flexible data input (such as the cell size of the grid and indicator’s weights) by resampling the territory, and its choice is open to the users. Each group of users would share their knowledge and arrive at a consensus for the data input.
After, an unsupervised machine learning algorithm is used to create the new taxonomy, but since the data input is open, the result of this taxonomy also is. K-means clustering has an early convergence point where the clusters are not re-organized at a specific number, but they start to create subcategories of one of the clusters. The way to choose the number of clusters is based on this convergence point. Several tests were performed by resampling the cell size, and scenario two was chosen because it allowed fewer clusters with a higher grid resolution.
SYSTEMIC RELATIONSHIPS & HYBRID APPROACH
Land use selectionAt this stage, land use is embedded in the analysis to propose practical territorial strategies. Arable land was chosen as a case study due to the lack of current proposals for these areas. At the same time, despite Vitoria being a benchmark city for sustainable development, only 4% of the agricultural land is currently considered sustainable.
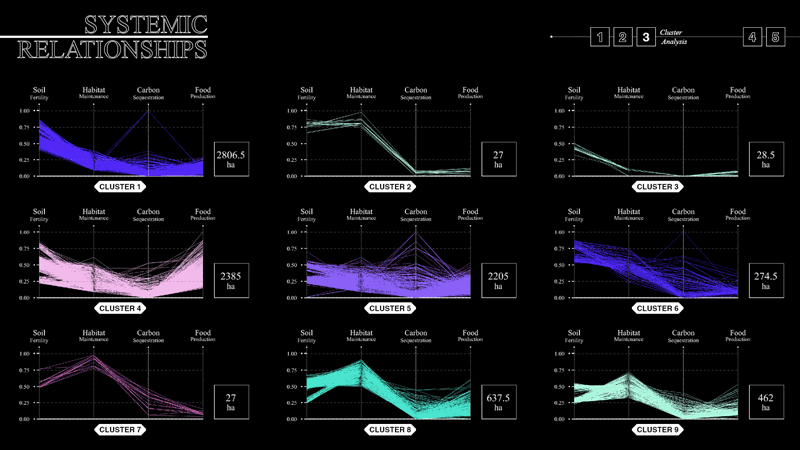
Cluster Analysis & hybrid strategies
This specific land use is composed of all the clusters of the taxonomy. We can conclude from the clustering that the relationship between the values creates the taxons. Each group represents an aggregation of similar relations regarding land’s multifunctionality, responding to a systemic understanding and not to the performance of one indicator. The modal value is calculated to avoid basing the cluster selection on performances. This value represents the most common values in the cluster. It allows having a representative image of the common trend of the cluster and being able to propose multiple strategies simultaneously.
Clusters are reordered based on similar trends of multifunctionality, where what matters is not the single modal value for each indicator but the curve that interconnects those values. The selected clusters show how food production in these specific areas has a significant trade-off in habitat maintenance and carbon sequestration. The objective of the hybrid strategies should focus on rebalancing the territory by changing these relationships. While in one cluster, food production could increase by intensifying agriculture while increasing soil fertility, the other clusters could reduce the food production in exchange for increased carbon sequestration and habitat maintenance.
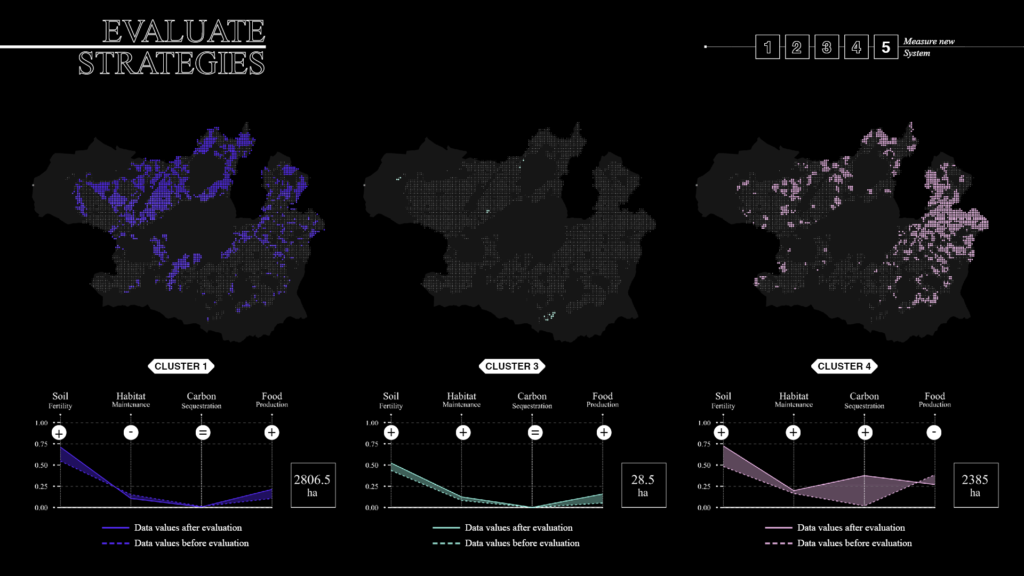
Trade-offs and synergies evaluation
At this stage, experts could evaluate the strategies by analyzing the new relational data values to understand which indicator is increased at the others’ expense.
RESPONSIVE TAXONOMY
With the evaluation, the last step of the methodology is accomplished, and therefore the strategies can be implemented. Once this implementation is applied gradually, a regular multifunctional land analysis can be redone again to monitor the strategies.
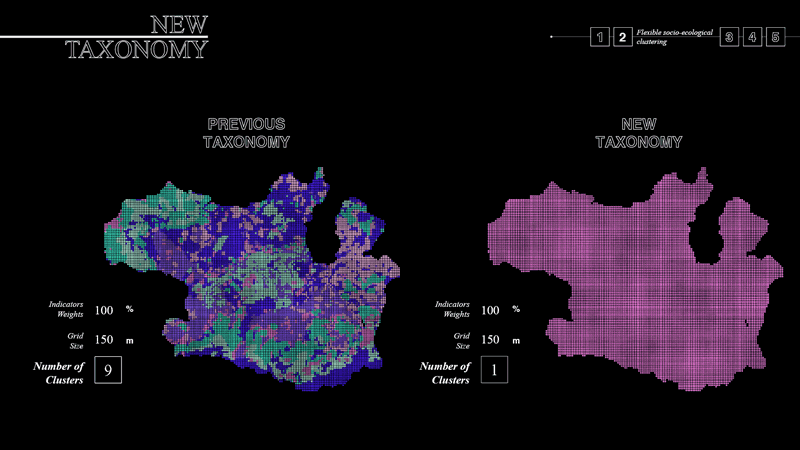
New taxonomy based on new data input
New data values mean new data input, which means a new taxonomy. Once the monitoring data enters the workflow, the clustering process restarts, and new taxons emerge. The feedback loop process, therefore, creates a responsive taxonomy based on the dynamic condition of the territory.
LIMITATIONS & CONCLUSIONS
It is needed to be acknowledged that this framework doesn’t take into account other forms of capital needed (such as machinery, amount of workers…) for the strategies to thrive apart from natural capital. For future steps, these measurements could be taken into account in order to be able to improve the decision-making process.
LUR represents the opportunity to create a framework based on territorial dynamics, where the constant feedback loop creates a continuous strategic process. There is no finalized strategy nor a static understanding of the land. In conclusion, LUR is a data-driven decision support system that adapts to the dynamics of land to allow transition towards resilience.
FUTURE STEPS: ama L.U.R.
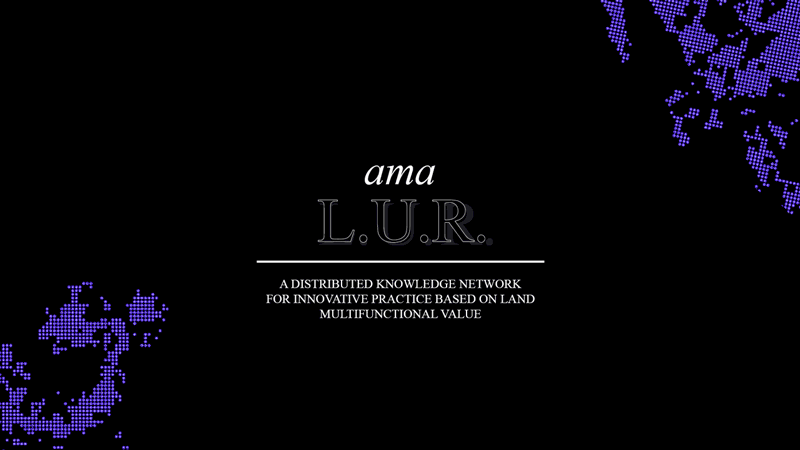
A distributed knowledge based network
L.U.R. framework can be replicated in similar regions in Europe, based on biophysical and socioeconomic similarities to empower a distributed knowledge network for innovative practice.In this network, each region could check for other regions and municipalities to see their strategies and percentage of implementation. The monitoring process could be achieved with a collaboration between top down measurements but also bottom up processes, where citizens could become data scientists while improving their knowledge on why land multifunctionality is important. Since the monitoring allows to measure the evolution of the progress, with the trade-offs and synergies of each of them, evidence based solutions can be consulted and replicated.
L.U.R.: A territorial decision support system for spatial development based on land’s multifunctional value is a project of IAAC, Institute for Advanced Architecture of Catalonia developed at Master in City & Technology in 2021/22 by student: Adriana Aguirre Such and faculty: Mathilde Marengo, Gonzalo Delacámara & Iacopo Neri